Forecasting accuracy plays a crucial role in decision-making across various industries, and one of the most reliable metrics for evaluating forecast accuracy is the Mean Absolute Scaled Error (MASE). MASE has become a standard tool for statisticians and data scientists because of its ability to provide a clear and interpretable measure of forecast performance. In this article, we'll explore the concept of MASE, its applications, and why it stands out compared to other forecasting error metrics.
MASE is particularly useful because it compares the forecast errors of a model to a naive forecast, offering a benchmark that's both intuitive and actionable. This makes it an essential metric for businesses, researchers, and analysts who rely on accurate predictions to drive strategy and growth.
Whether you're working in finance, supply chain management, or any field that requires predictive analytics, understanding MASE can help you make more informed decisions. In the sections below, we will delve into the technical aspects of MASE, its advantages, and practical examples of its use in real-world scenarios.
Table of Contents
- Introduction to MASE
- How MASE Works
- Advantages of Using MASE
- Comparison with Other Metrics
- Applications of MASE
- Mathematical Formulation
- Interpreting MASE Values
- Real-World Examples
- Common Mistakes When Using MASE
- Conclusion and Next Steps
Introduction to MASE
The Mean Absolute Scaled Error (MASE) was introduced by Rob J. Hyndman and Anne B. Koehler in 2006 as a robust way to evaluate forecasting accuracy. Unlike traditional metrics such as Mean Absolute Error (MAE) or Root Mean Square Error (RMSE), MASE provides a normalized measure that makes it easier to compare models across different datasets.
Why is MASE Important?
MASE addresses one of the key limitations of other metrics: the lack of a meaningful benchmark. By comparing forecast errors to a naive model, MASE ensures that the performance of any forecasting model is assessed against a realistic baseline. This normalization is particularly valuable when working with time series data that exhibit seasonality or trends.
For instance, a MASE value less than 1 indicates that the model performs better than the naive forecast, while a value greater than 1 suggests that the model is underperforming. This simple interpretation makes MASE an indispensable tool for anyone involved in predictive analytics.
How MASE Works
MASE operates by scaling the forecast errors using the Mean Absolute Error (MAE) of a naive forecast. The naive forecast assumes that the next period's value will be the same as the previous period's value. This baseline provides a fair comparison point for evaluating the accuracy of more sophisticated models.
Steps in Calculating MASE
- Compute the forecast errors for the model being evaluated.
- Calculate the MAE of the naive forecast.
- Divide the model's MAE by the naive forecast's MAE to obtain the scaled error.
This process ensures that the results are normalized and comparable across different datasets, making MASE a versatile metric for forecasting evaluation.
Advantages of Using MASE
MASE offers several advantages over other forecasting error metrics:
1. Intuitive Interpretation
MASE provides a straightforward interpretation of forecast accuracy. A value of 1 means the model performs as well as the naive forecast, while values below 1 indicate superior performance.
2. Robustness
MASE is less sensitive to outliers compared to metrics like RMSE, making it a more reliable choice for datasets with irregularities.
3. Normalization
By scaling errors against the naive forecast, MASE ensures that comparisons between models and datasets are fair and meaningful.
Comparison with Other Metrics
While MASE is a powerful tool, it's essential to understand how it compares to other commonly used metrics:
Mean Absolute Error (MAE)
MAE measures the average magnitude of errors in a set of predictions. However, it lacks the normalization provided by MASE, making it less suitable for cross-dataset comparisons.
Root Mean Square Error (RMSE)
RMSE emphasizes larger errors due to its quadratic nature, which can skew results in datasets with outliers. MASE, on the other hand, offers a more balanced evaluation.
Mean Absolute Percentage Error (MAPE)
MAPE expresses errors as percentages, but it can be problematic when actual values are close to zero. MASE avoids this issue by using a scaled error approach.
Applications of MASE
MASE finds applications in various fields where forecasting is critical:
1. Finance
In financial markets, accurate forecasts of stock prices, exchange rates, and economic indicators are essential. MASE helps analysts evaluate the performance of predictive models in this domain.
2. Supply Chain Management
Forecasting demand for products is crucial for optimizing inventory levels and reducing costs. MASE enables supply chain professionals to assess the accuracy of their demand forecasting models.
3. Energy Sector
Energy companies rely on forecasts to predict electricity consumption and plan production accordingly. MASE provides a reliable metric for evaluating the accuracy of these forecasts.
Mathematical Formulation
The mathematical formula for MASE is as follows:
MASE = mean(|et|) / mean(|qt|)
Where:
- et = forecast error at time t
- qt = naive forecast error at time t
This formulation ensures that the results are normalized and comparable across different datasets.
Interpreting MASE Values
Understanding MASE values is key to leveraging this metric effectively:
1. Values Less Than 1
A MASE value below 1 indicates that the model outperforms the naive forecast, suggesting that it is a good choice for the given dataset.
2. Values Greater Than 1
A MASE value above 1 implies that the model performs worse than the naive forecast, indicating the need for improvement or alternative approaches.
3. Value of 1
A MASE value of exactly 1 means the model performs as well as the naive forecast, which may not be sufficient for practical applications.
Real-World Examples
Let's explore some real-world examples where MASE has been successfully applied:
1. Retail Sales Forecasting
A retail company used MASE to evaluate the accuracy of its sales forecasting models. By comparing different models using MASE, they identified the most effective approach, leading to improved inventory management and increased profitability.
2. Weather Forecasting
Meteorologists employ MASE to assess the accuracy of weather prediction models. This ensures that forecasts are reliable and actionable, helping to mitigate the impact of severe weather events.
3. Healthcare Demand Forecasting
Hospitals use MASE to evaluate models predicting patient admissions. Accurate forecasts enable better resource allocation and improved patient care.
Common Mistakes When Using MASE
While MASE is a powerful tool, there are common pitfalls to avoid:
1. Overlooking Dataset Characteristics
MASE assumes that the data is stationary or has been appropriately transformed. Failing to account for non-stationarity can lead to misleading results.
2. Ignoring Model Complexity
More complex models may yield lower MASE values but could suffer from overfitting. It's important to balance model complexity with generalization ability.
3. Misinterpreting Results
MASE values should be interpreted in context. A value slightly above 1 may still be acceptable depending on the application and dataset.
Conclusion and Next Steps
In conclusion, MASE is a valuable metric for evaluating forecast accuracy due to its normalization, robustness, and intuitive interpretation. Whether you're working in finance, supply chain management, or any other field that relies on predictive analytics, understanding and applying MASE can significantly enhance your decision-making capabilities.
We encourage you to explore MASE further and apply it to your forecasting challenges. Feel free to leave a comment below sharing your experiences or questions. Additionally, don't forget to explore other articles on our site for more insights into data science and forecasting techniques.
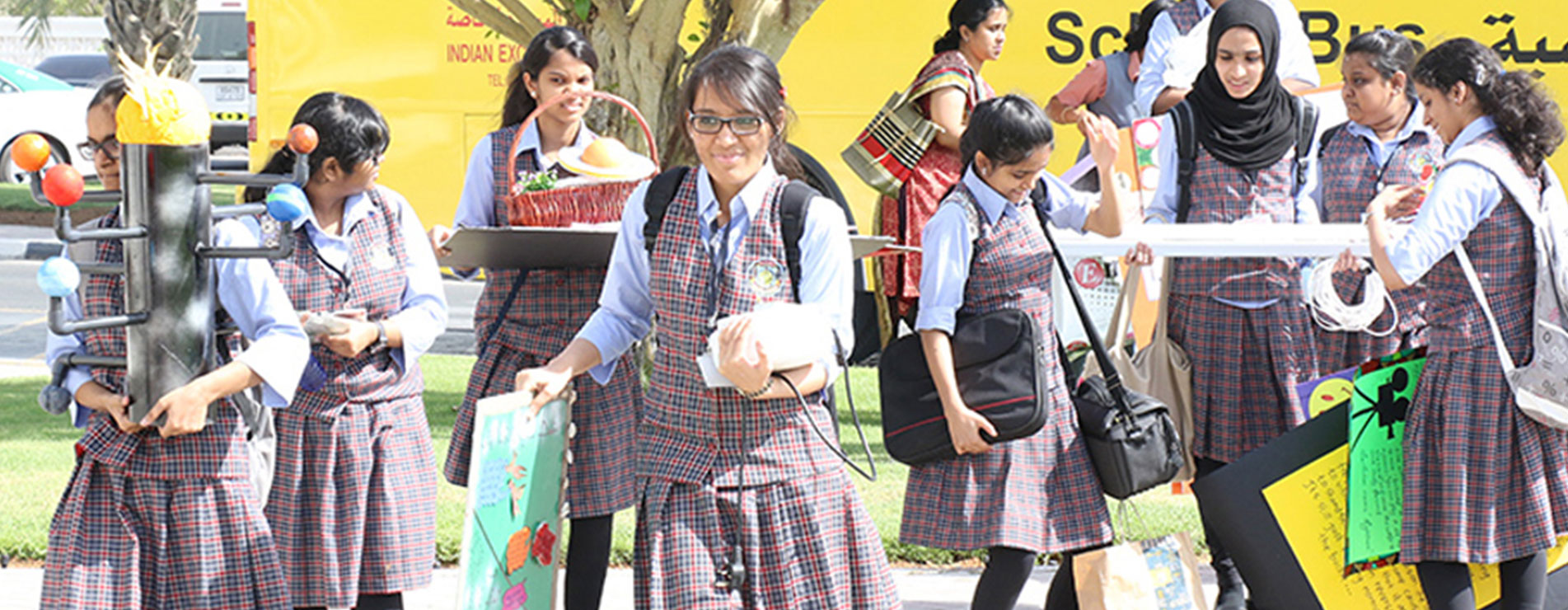
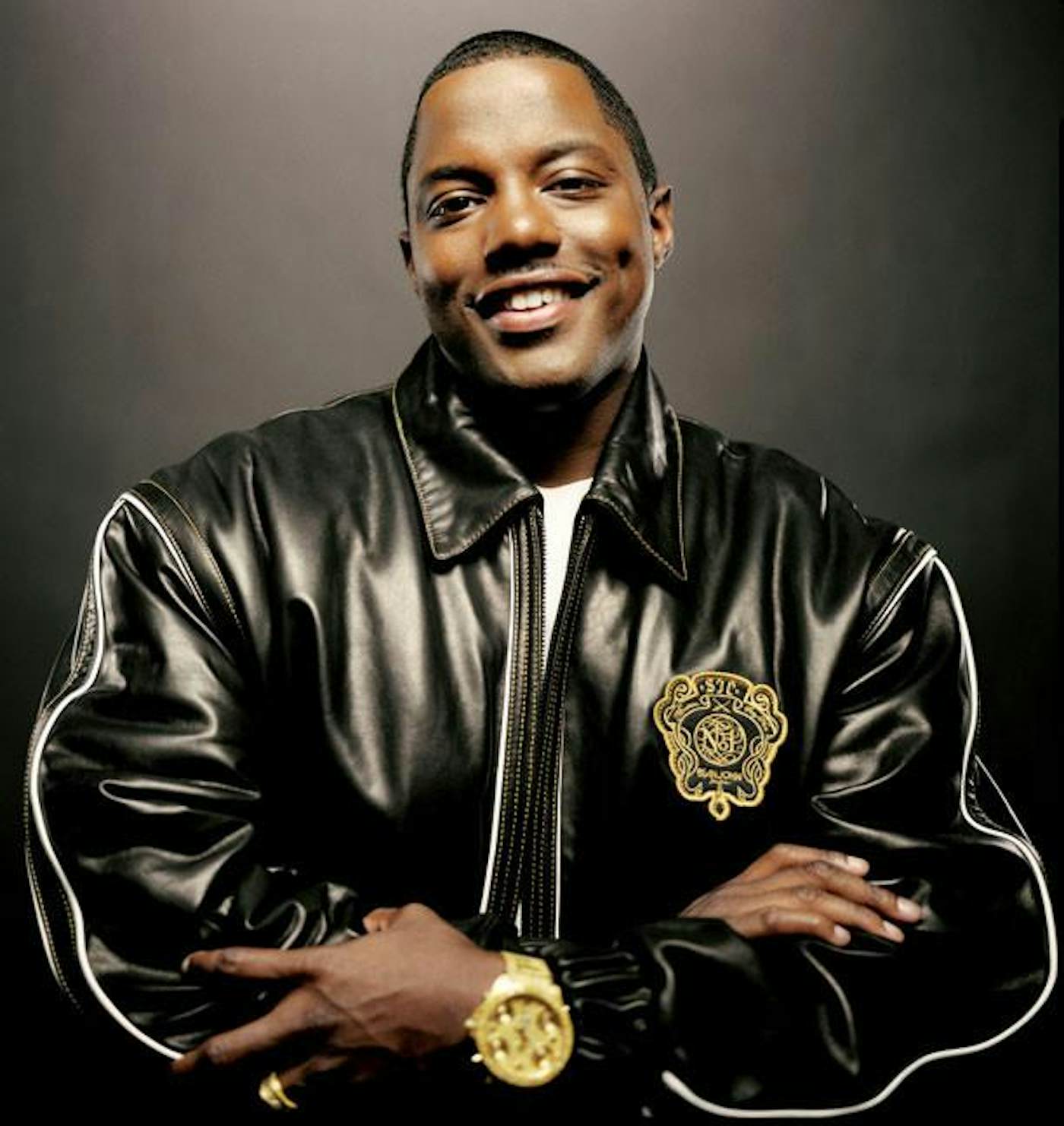
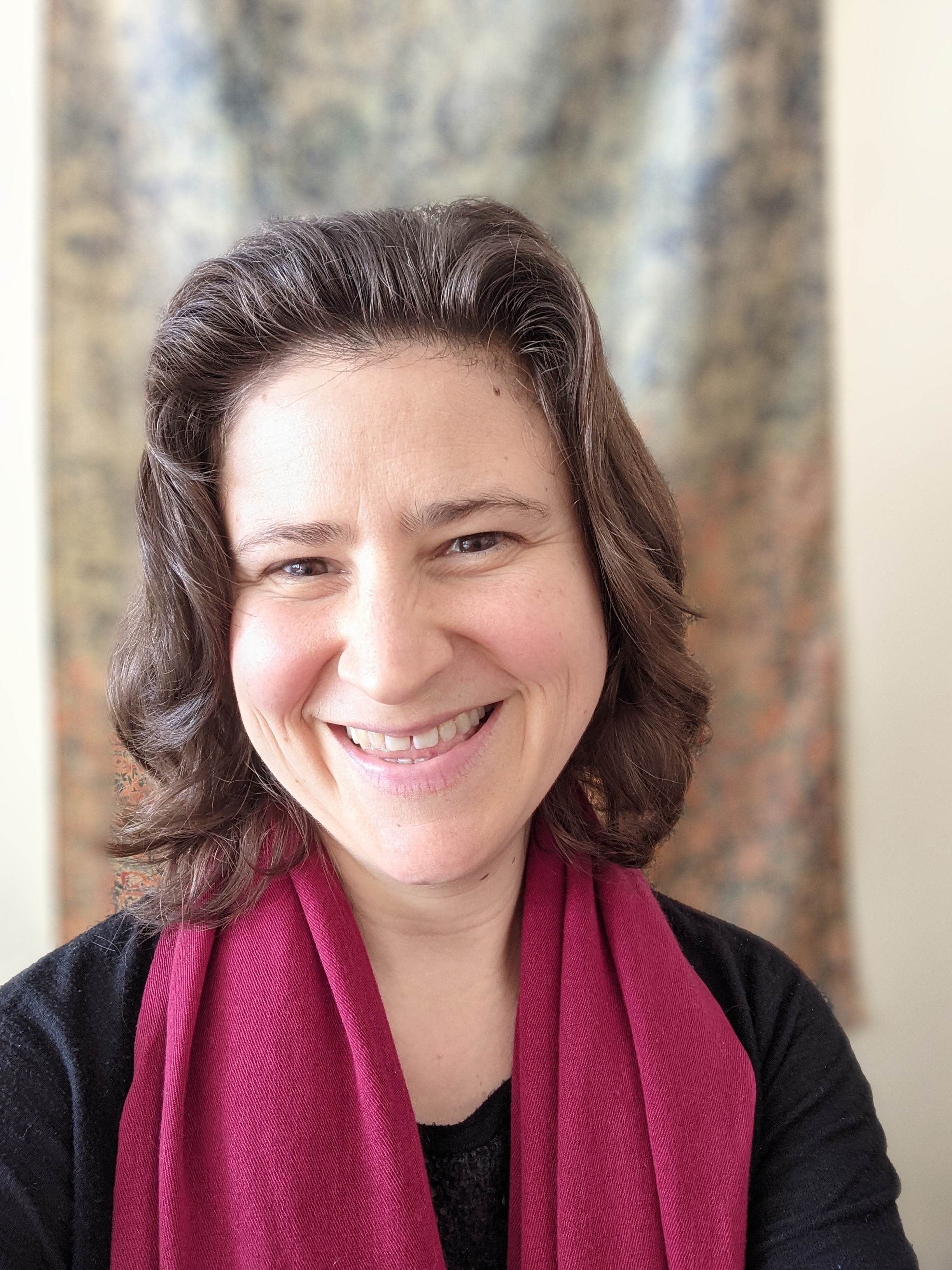